Simultaneous Linear Equations- Guass-Jorda Elimination
- Backsubstitution
- Pivoting
- LU Decomposition
- Inverse of a Matrix
- Tridagonal and banded Matrices
Eigenvalues and Eigenvectors
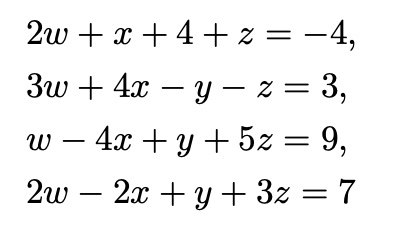
with matrix form,
represent with Matrix A, and vector x, v
achive sol by inverse matrix
Gauss-Jordan Elimination
rules for Gauss-Jordan Elimination
1. if we multiply any row of the matrix A by any constant,
and we multiply the correponding row of the vector v by the same constant,
then the solution does not change.
2. if we add to or substract from any row of A a mltiple of any other row, and we do the
same for the vector v, then the solutio ndoes not change.
after Gauss-Jordan Elimination achive "Upper Diagonal Matrix”
Backsubstitution
can be written as,
x_i=v_i-sigma( {j=i+1}^{n-1}(a_ij*x_j) ) <- x_i는 역순으로 구해야 함
from numpy as np
A=np.array([[2, 1, 4, 1], [3, 4, -1, -1], [1, -4, 1, 5], [2, -2, 1, 3]], float)
v=np.array([-4, 3, 9, 7], float)
N=A.shape[0]; M=A.shape[1]
x=np.zeros([4, 1], float)
for n in range(N):
div=A[n, n]
A[n, :]/=div
v[n]/=div
for i in range(n+1, N):
mult=A[i, n]
A[i, :]-=mult*A[n, :]
v[i]-=mult*v[n]
for i in range(N-1, -1, -1):
x[i]=v[i]
for j in range(i+1, N):
x[i]-=A[i, j]*x[j]
print("solution x=\n", x, sep="")
Pivotingfirst element가 zero인 경우(Divide by zero is not allowed)
일반적으로 0과 가장 거리가 먼 행과 변경
Partial Pivoting
import numpy as np
A=np.array([[0, 1, 4, 1], [3, 4, -1, -1], [1, -4, 1, 5], [2, -2, 1, 3]], float)
v=np.array([-4, 3, 9, 7], float)
N=A.shape[0]; M=A.shape[1]
x=np.zeros([4, 1], float)
for n in range(N):
max_el=abs(A[n, n])
loc_max_el=n
for j in range(n+1, N):
if abs(A[j, n])>max_el:
max_el=abs(A[j, n])
loc_max_el=j
if loc_max_el!=n:
A_tmp=A[n, :].copy()
A[n, :]=A[loc_max_el, :]
A[loc_max_el, :]=A_tmp
v_tmp=v[n].copy()
v[n]=v[loc_max_el]
v[loc_max_el]=v_tmp
div=A[n, n]
A[n, :]/=div
v[n]/=div
for i in range(n+1, N):
mult=A[i, n]
A[i, :]-=mult*A[n, :]
v[i]-=mult*v[n]
for i in range(N-1, -1, -1):
x[i]=v[i]
for j in range(i+1, N):
x[i]-=A[i, j]*x[j]
print("solution x=\n", x, sep="")
Gauss-Jordan Elimination in Matrix Form Repeating Gauss-Jordan elimination would be time-consuming(역행렬 연산에서 연산량 큼)
step 1:
Define lower triangular matrix L_0 as,
step 2:
Define lower trianuglar matrix L_1 as,
step 3:
Define lower trianuglar matrix L_2 as,
step 4:
=

Define lower trianuglar matrix L_3 as,
Dfine two matrices:
(L is Lower triangular matrix, U is Upper triangle matrix)
then,
from A^-1.dot(A)= I (Identity)
LU Decomposition-Backsubtraction
express with 3x3 matrix A
with Ax=v
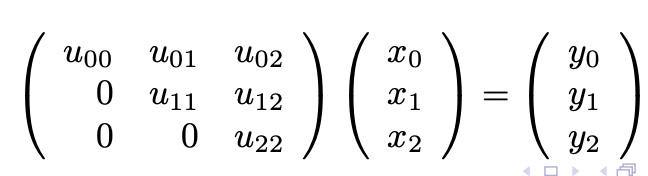
with y,
y satisfied,
then,
매 단계마다 Paritial Pivoting 필요
from numpy.linalg import solve
x=solve(A, v)
LU decomposition with partial pivoting
import numpy as np
A=np.array([[0, 1, 4, 1], [3, 4, -1, -1], [1, -4, 1, 5], [2, -2, 1, 3]], float)
v=np.array([-4, 3, 9, 7], float)
N=A.shape[0]; M=A.shape[1]
x=np.zeros([N, 1], float)
L=np.zeros_like(A)
U=np.copy(A)
for n in range(N):
max_el=abs(U[n, n])
loc_max_el=n
for j in range(n+1, N):
if abs(U[j, n])>max_el:
max_el=abs(U[j, n])
loc_max_el=j
if loc_max_el!=n:
U_tmp=U[n, :].copy()
U[n, :]=U[loc_max_el, :]
U[loc_max_el, :]=U_tmp
L_tmp=L[n, :].copy()
L[n, :]=L[loc_max_el, :]
L[loc_max_el, :]=L_tmp
A_tmp=A[n].copy()
A[n]=A[loc_max_el]
A[loc_max_el]=A_tmp
v[n], v[loc_max_el]=v[loc_max_el], v[n]
L[n:, n]=U[n:, n]
div=U[n, n]
U[n, :]/=div
for i in range(n+1, N):
mult=U[i, n]
U[i, :]-=mult*U[n, :]
print("A=", A)
print("LU=", np.dot(L, U))
y=np.zeros_like(v, float)
for i in range(M):
y[i]=v[i]
for j in range(0, i):
y[i]-=L[i, j]*y[j]
y[i]/=L[i, i]
for i in range(M-1, -1, -1):
x[i]=y[i]
for j in range(i+1, M):
x[i]-=U[i, j]*x[j]
print("solution x=\n", x, sep="")
Calculating the Inverse of a Matrixseperate with column
n 번의 Gauss-Jordan elimination or LU decompositon을 이용해서 해를 구할 수 있음
V=I로 둘경우, X가 A의 inverse matrix가 됨
from numpy.linalg import inv
X=inv(A)
Inverse Matrix
import numpy as np
A=np.array([[0, 1, 4, 1], [3, 4, -1, -1], [1, -4, 1, 5], [2, -2, 1, 3]], float)
N=A.shape[0]; M=A.shape[1]
L=np.zeros_like(A)
U=np.copy(A)
V=np.diag([1, 1, 1, 1])
for n in range(N):
max_el=abs(U[n, n])
loc_max_el=n
for j in range(n+1, N):
if abs(U[j, n])>max_el:
max_el=abs(U[j, n])
loc_max_el=j
if loc_max_el!=n:
U_tmp=U[n, :].copy()
U[n, :]=U[loc_max_el, :]
U[loc_max_el, :]=U_tmp
L_tmp=L[n, :].copy()
L[n, :]=L[loc_max_el, :]
L[loc_max_el, :]=L_tmp
V_tmp=V[n, :].copy()
V[n, :]=V[loc_max_el, :]
V[loc_max_el, :]=V_tmp
L[n:, n]=U[n:, n]
div=U[n, n]
U[n, :]/=div
for i in range(n+1, N):
mult=U[i, n]
U[i, :]-=mult*U[n, :]
print("LU=", np.dot(L, U))
Y=np.zeros_like(V, float)
X=np.zeros_like(V, float)
for i in range(N):
for j in range(N):
Y[j, i]=V[j, i]
for k in range(j):
Y[j, i]-=L[j, k]*Y[k, i]
Y[j, i]/=L[j, j]
for i in range(N):
for j in range(N-1, -1, -1):
X[j, i]=Y[j, i]
for k in range(j+1, N):
X[j, i]-=U[j, k]*X[k, i]
X[j, i]/=U[j, j]
print("X=", X)
print("I=", A.dot(X))
Tridiagonal Matrices: Trigonal Matrix Algorithm or Thomas AlgorithmMatrix has nonzero elements only along the diagonal and immediately above and below it
with Gauss Jordan Elimination(make Trigonal Matrix)
Backsubstitution
Banded Matrix
triangular Matrix는 더 간단하게 Gauss Jordan Elimination 가능
Vibration in a One-Dimensional SystemThe masses at the two end
assuming,
and
then,
->
with
set m=1, k=6, w=2
import numpy as np
import matplotlib.pyplot as plt
N=26
C=1.0
m=1.0
k=6.0
omega=2.0
alpha=2*k-m*omega**2
A=np.zeros([N, N])
for i in range(N-1):
A[i, i]=alpha
A[i, i+1]=-k
A[i+1, i]=-k
A[0, 0]-=k
A[N-1, N-1]=alpha-k
v=np.zeros(N, float)
v[0]=C
for i in range(N-1):
div=A[i, i]
A[i, i]/=div
A[i, i+1]/=div
v[i]/=div
if i==N-2:
n=2
else:
n=3
a_tmp=A[i+1, i]
for j in range(n):
A[i+1, i+j]-=A[i, i+j]*a_tmp
v[i+1]-=a_tmp*v[i]
A[i+1, i]=0
v[N-1]/=A[N-1, N-1]
A[N-1, N-1]/=A[N-1, N-1]
x=np.zeros_like(v, float)
x[N-1]=v[N-1]
for i in range(N-2, -1, -1):
x[i]=v[i]-A[i, i+1]*x[i+1]
plt.plot(x)
plt.plot(x, "ko", ms=15.0)
plt.plot(xx, "rs")
plt.show()
more optimized(Teoplitz-Trigonal matrix only)
import numpy as np
import matplotlib.pyplot as plt
N=26
C=1.0
m=1.0
k=6.0
omega=2.0
alpha=2*k-m*omega**2
A=np.zeros([N, N])
for i in range(N-1):
A[i, i]=alpha
A[i, i+1]=-k
A[i+1, i]=-k
A[0, 0]-=k
A[N-1, N-1]=alpha-k
v=np.zeros(N, float)
v[0]=C
for i in range(N-1):
div=A[i, i]
A[i, i]/=div
A[i, i+1]/=div
v[i]/=div
mul=A[i+1, i]
A[i+1, i+1]-=mul*A[i, i+1]
v[i+1]-=mul*v[i]
A[i+1, i]=0
v[N-1]/=A[N-1, N-1]
A[N-1, N-1]/=A[N-1, N-1]
x=np.zeros_like(v, float)
x[N-1]=v[N-1]
for i in range(N-2, -1, -1):
x[i]=v[i]-A[i, i+1]*x[i+1]
plt.plot(x)
plt.plot(x, "ko", ms=15.0)
plt.plot(xx, "rs")
plt.show()
Eigenvalues and Eigenvectorsin physics
- Mechanics
- Electromagnetism
- Quantum mechanism
- etc.
only focus on real Symmetric matrix A
for Symmetric matrix A’s eigenvectors are orthogonal
QR decomposition to eigenvectors, eigenvalues
from eigen decomposition
because V is Orthogonal matrix
rewrite the matrix A as product QR
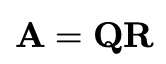
Q: an orthogonal matrix, R: Upper-triangular matrix
and define
then,
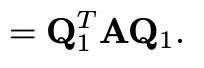
set,
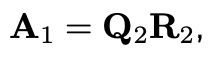
then,
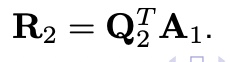
and define
Repeat the process up to total k steps then,
process long enough, matrix A_k become diagonal.
set,
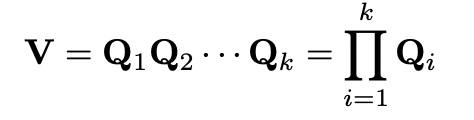
same as eigen decomposition
QR decomposition
1. Create NxN matrix V to hold the eigenvectors
2. Initialize V to be equal to the identity matrix I
3. Choose a target accuracy for off-diagonal elements of the eigenvalue matrix
4. Calculate the QR decomposition A=QR
5. Update A to the new value A=RQ
6. Multiply V on the right by Q
7. Check the off-diagonal elemetns of A. If they are all less than error, we are done
otherwise go back to step 4
import numpy as np
V=np.linalg.eigh(A)
lamb=np.linalg.eigvalsh(A)
then, how to QR decompositionwith A
Gram-Schmidt Orthogonalization
then,
eigenvectors & eigenvalues
import numpy as np
A=np.array([[1, 4, 8, 4], [4, 2, 3, 7], [8, 3, 6, 9], [4, 7, 9, 2]], float)
xx, VV=np.linalg.eigh(A)
N=A.shape[1]
U=np.zeros_like(A, float)
Q=np.zeros_like(A, float)
R=np.zeros_like(A, float)
diag=np.ones(N, float)
V=np.diag(diag)
delta=1.0
epsilon=1.e-10
while delta>epsilon:
for i in range(N):
U[:, i]=A[:, i]
if i>0:
for j in range(i):
U[:, i]-=(np.dot(Q[:, j], A[:, i])*Q[:, j])
magU=np.dot(U[:, i], U[:, i])**(1/2)
Q[:, i]=U[:, i]/magU
for j in range(N):
for k in range(N):
if j>k:
R[j, k]=0
elif j==k:
R[j, k]=np.dot(U[:, j], U[:, j])**(1/2)
else:
R[j, k]=np.dot(Q[:, j], A[:, k])
A=np.dot(R, Q)
V=np.dot(V, Q)
delta=0.0
for j in range(N):
for k in range(N):
if j<k:
if delta<abs(A[j, k]):
delta=abs(A[j, k])
lamb=np.zeros(N, float)
for i in range(N):
lamb[i]=A[i][i]